Lung cancer remains one of the leading causes of cancer-related deaths globally, with an estimated 1.8 million deaths in 2020 alone. Early detection is crucial for improving survival rates, and artificial intelligence (AI) is emerging as a game-changer in this field. By harnessing advanced algorithms and machine learning techniques, AI is revolutionizing how we predict and diagnose lung cancer, offering new hope for patients and healthcare providers alike.
The Power of AI in Lung Cancer Prediction
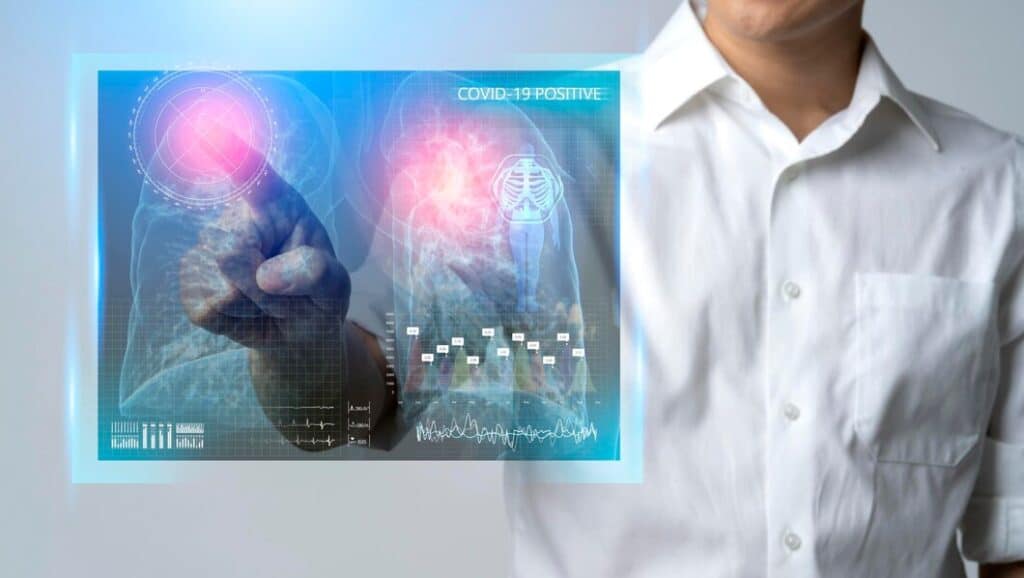
AI models are increasingly being utilized to enhance lung cancer screening processes. These models analyze vast amounts of data from various sources, including imaging studies, electronic health records, and patient demographics, to identify individuals at high risk for developing lung cancer. The integration of AI into clinical practice allows for more accurate predictions and timely interventions.
Key Applications of AI in Lung Cancer Detection
- Imaging Analysis: AI algorithms excel at analyzing medical images such as low-dose CT scans and chest radiographs. By employing deep learning techniques, these systems can detect suspicious nodules with remarkable accuracy. For instance, convolutional neural networks (CNNs) have demonstrated high sensitivity in identifying malignant nodules, significantly enhancing early detection rates.
- Risk Assessment Models: Recent advancements have led to the development of simplified risk prediction models that require minimal input data. Researchers from University College London (UCL) created a model that predicts lung cancer risk using just three variables: age, smoking history, and average daily cigarette consumption. This approach not only improves accessibility but also streamlines the data collection process.
- Integration of Biomarkers: AI can analyze both imaging data and biological markers to refine risk assessments further. By combining traditional clinical data with advanced imaging techniques, AI models can provide a comprehensive view of a patient’s risk profile, leading to personalized screening strategies.
- Predictive Analytics: Machine learning models have shown promise in predicting outcomes based on historical data. For example, studies have demonstrated that AI can accurately forecast survival rates based on tumor characteristics and patient demographics, aiding in treatment planning and decision-making.
- Reducing False Positives: One significant challenge in lung cancer screening is the high rate of false positives that can lead to unnecessary anxiety and invasive procedures. AI algorithms help mitigate this issue by improving specificity—ensuring that benign nodules are less likely to be misclassified as malignant.
Challenges Ahead
Despite the promising advancements in AI-driven lung cancer prediction models, several challenges remain. Ensuring the accuracy and reliability of these models across diverse populations is crucial for their widespread adoption. Additionally, integrating AI systems into existing healthcare infrastructures requires careful consideration of ethical implications, data privacy concerns, and clinician training.
Conclusion
AI’s role in early lung cancer detection represents a significant leap forward in oncology. By enhancing imaging analysis, simplifying risk assessments, and integrating biological markers into predictive models, AI is poised to transform how we approach lung cancer screening and diagnosis. As research continues to evolve and clinical applications expand, the potential for AI to save lives through early detection becomes increasingly tangible.
FAQs
- How does AI improve lung cancer detection?
AI enhances detection by analyzing medical images and patient data more accurately than traditional methods, identifying suspicious nodules that may indicate cancer. - What are the key variables used in AI risk prediction models?
Recent models have successfully predicted lung cancer risk using just three variables: age, smoking history, and average daily cigarette consumption. - Can AI reduce the number of false positives in lung cancer screenings?
Yes, AI algorithms improve specificity in detecting malignant nodules, thereby reducing the incidence of false positives associated with traditional screening methods. - What types of data do AI models analyze for lung cancer prediction?
AI models analyze a combination of imaging studies (like CT scans), electronic health records, clinical parameters (e.g., symptoms), and biomarkers to assess risk accurately. - Are there any limitations to using AI for lung cancer detection?
Limitations include ensuring model accuracy across diverse populations and addressing ethical concerns related to data privacy and algorithm transparency. - What does the future hold for AI in lung cancer detection?
The future looks promising as ongoing research aims to validate AI models further and integrate them into routine clinical practice for enhanced early detection.